Ever since Google announced its Knowledge Graph solution in 2012 the paradigm has found its way into many real-world use cases, mostly in the analytics space. This presentation will cover what a Knowledge Graph is, how it is different and yet complementary and will look at vendors, products and standards.
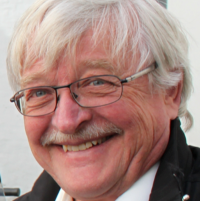